Detection of Duplicate Records in Large-scale Multi-tenant Platforms
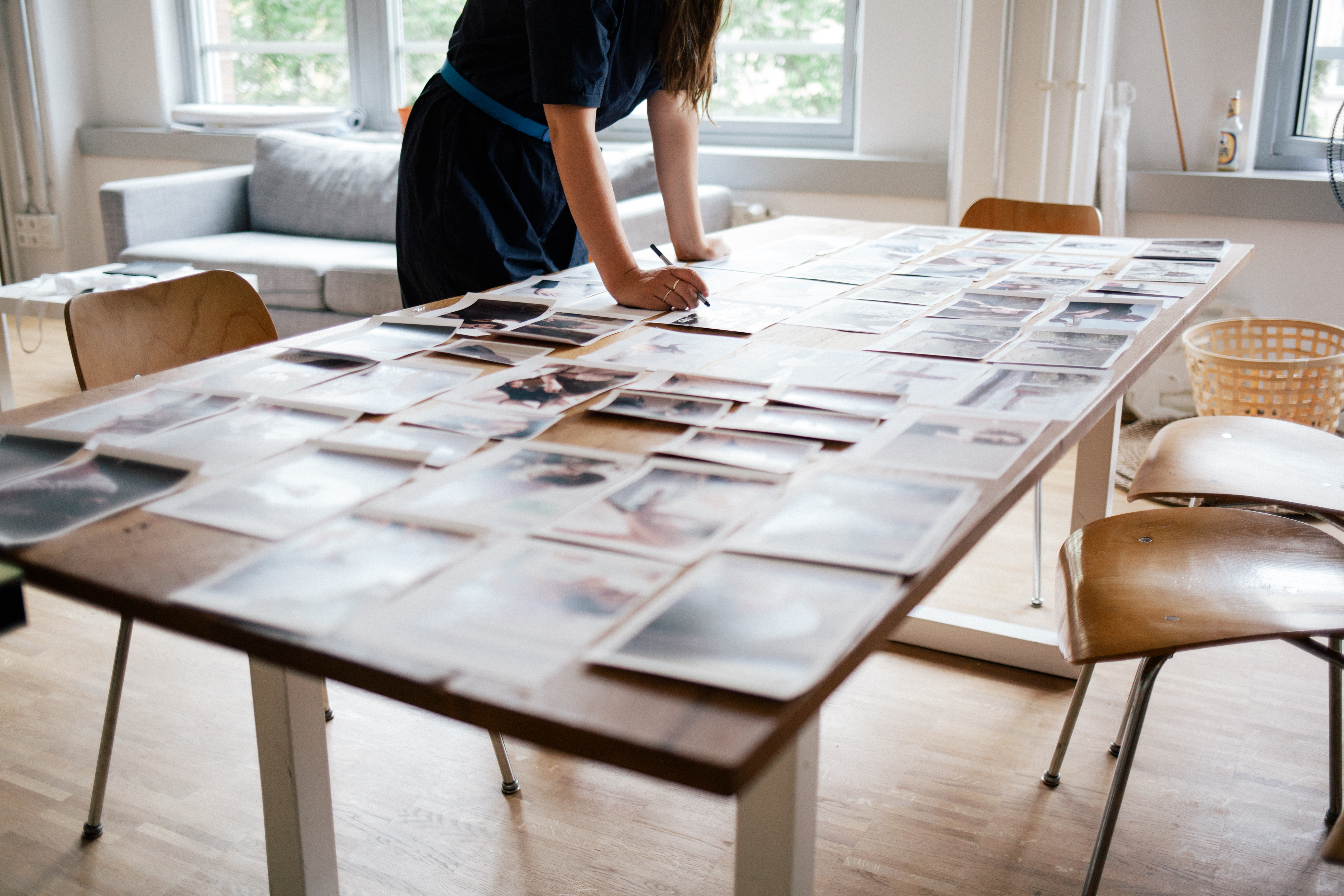
Paul O'Grady
#PyConIE - 6th November 2016
Paul O'Grady
#PyConIE - 6th November 2016
Currently Data Scientist at ...
Twitter: @paul_ogrady
The task of finding records in a data set that refer to the same entity across different data sources
Necessary when joining data sets based on entities that may or may not share a common identifier—canonical ID
Common problem, e.g.:
+----+------------+-------------+---------------------------------+
| ID | First Name | Second Name | Address |
+----+------------+-------------+---------------------------------+
| 19 | G. | Van Rossum | PSF, 9450 SW Gemini Dr. ... |
+----+------------+-------------+---------------------------------+
| 56 | Guido | V. Rossum | Python Software Foundation, ... |
+----+------------+-------------+---------------------------------+
Records \(\equiv\) Products
We will be looking at Categorical Data
Products exist in space/manifold
Distances between products indicate similarity
Product duplicates are products that are very similar
Cartesian Join / All-pairs Join / Similarity Join \(O(n^2)\) *
Naive Solution:
sim_scores = []
for i, record_i in enumerate(records):
for j, record_j in enumerate(records):
sim_scores.append(((i, j), sim(record_i, record_j)))
Output:
>>> sim_scores
[((0, 0), 0), ((0, 1), 4), ((0, 2), 0), ((1, 0), -4), ((1, 1), 0),
((1, 2), -4), ((2, 0), 0), ((2, 1), 4), ((2, 2), 0)]
* To be more correct our problem is O(n(n-1)/2)
How to calculate sim(record_i, record_j)
?
Composite Similarity Measure:
Weighted combination of individual attribute similarity measures, \({f_i,\dots,f_N}\):
where \({a_i,\dots,a_N}\) are the product attributes, \({f_i,\dots,f_N}\) are their similarity measures and \({w_i,\dots,w_N}\) are the weighting in the model [1].
\(f_i\) we use Hamming Distance between attributes *
\(w_i\) are learned from training data using an Averaged Perceptron, \(\hat y = \text{sign}(\mathbf{w_{avg}} \cdot \mathbf{x})\)
Code:
for t in range(T):
for i in range(M):
x = training_data[i,:] # f() applied in advance
y = labels[i]
dot_prod = float(w.dot(np.matrix(x).T))
y_est = sgn(dot_prod)
if y_est != y:
w = w + (y * x)
w_avg += w
w_avg = w_avg / (T*M)
* We use other categorical similarity measures that we do not discuss here
The Hamming/Overlap distance between two records is the number of attributes in which they differ
Code:
def hamming_distance(x, y):
return sum(elx != ely for elx, ely in zip(x, y))
Output:
>>> x = "Foo Bar Baz Qux"; y = "Foo Bar Baz corge"
>>> hamming_distance(x, y)
1
Q: If we relax our requirements on missing duplicates can we use a trick?
A: Yes - Locality-Sensitive Hashing (LSH)
LSH hashes input items so that similar items map to the same buckets with high probability [2]
LSH differs from cryptography hash functions because it aims to maximize the probability of collisions
Similarity is measured using the Jaccard Index
LSH parameters:
LSH is an exercise in tuning r & b for desired similarity & accuracy
The Jaccard index is useful for comparing segments of text
Code:
def jaccard_index(x, y):
return len(x.intersection(y)) / len(x.union(y))
Example:
>>> x = "Foo Bar Baz Qux"; y = "Qux Baz Bar Quux"
>>> jaccard_index(set(x.split()), set(y.split()))
0.6
Simple hashing function that hashes \(x\) to \(N\) buckets:
where \(a\) is a random integer & \(N\) is a prime number (to avoid collisions)
Code:
class SimpleHash:
def __init__(self, a, N, c=1):
self.a = a; self.N = N; self.c = c
def __call__(self, x):
return (self.a * x + self.c) % self.N
Example:
>>> hash_ = SimpleHash(a=5, N=7)
>>> [hash_(i) for i in range(7)]
[1, 6, 4, 2, 0, 5, 3]
Convert large sets to short signatures, while preserving similarity
For a random permutation \(\pi\).
Code:
def min_hash(a, hash_func):
perm_ind = [hash_func(x) for x in range(a.shape[0])]
return np.ma.masked_array(perm_ind, np.logical_not(a)).min()
Example:
>>> record_elements = np.array([0, 0, 1, 1, 0, 1, 1] )
>>> hash_ = simple_hash(a=5, N=7); [hash_(i) for i in range(7)]
[1, 6, 4, 2, 0, 5, 3]
>>> min_hash(record_elements, hash_)
2
For s-similar products, where r is the number of rows and b is the number of bands
prob. that all rows in band equal, \(t^r\)
prob. that some row in band unequal, \(1 - t^r\)
prob. that no band identical, \((1 - t^r)^b\)
prob. that at least 1 band identical (s-curve),
LSH params: \(r=4\) & \(b=16\). Candidate Pair Probability:
Similarity Probability 0.00 0.000000000 0.10 0.001598801 0.20 0.025295082 0.30 0.122016723 0.40 0.339616210 0.50 0.643925870 0.60 0.891481916 0.70 0.987637591 0.80 0.999782058 0.90 0.999999962
Optimise \(1 - (1 - t^r)^b\)
How to optimise?
sympy
& scipy
sympy
class SCurveOpt:
# Opt. *s*-curve Threshold
def candidate_pair_prob(self, r, b, s):
return 1 - (1 - s**r)**b
def candidate_pair_prob_derivative(self, r, b, s):
s_sym = Symbol('s')
y = self.candidate_pair_prob(r, b, s_sym)
yprime = y.diff(s_sym)
f = lambdify(s_sym, yprime, 'numpy')
return f(s)
def scurve_threshold(self, r, b):
x0 = (1/b)**(1/r)
func = lambda s :-self.candidate_pair_prob_derivative(r, b, s)
res = minimize(func, x0, method='L-BFGS-B',bounds=[(0.,1.)])
return res.x
# Opt. Area Under Curve
def candidate_pair_prob_integral(self, r, b, lower, upper):
s_sym = Symbol('s')
return float(Integral(self.candidate_pair_prob(s_sym, b, r),
(s_sym, lower, upper)))
def calc_false_positive_pct_area(self, r, b):
total_area = self._candidate_pair_prob_integral(r, b, 0., 1.)
area_left_of_threshold = self._candidate_pair_prob_integral(
r, b, 0, self.scurve_threshold_)
return area_left_of_threshold / total_area
# Opt. for Both
def __call__(self, r, b):
self.scurve_threshold_ = self.scurve_threshold(r, b)
self.calc_false_positive_area_ = \
self.calc_false_positive_pct_area(r, b)
self.scurve_threshhold_error_ = \
abs(self.scurve_threshold_ - self.target_s_thresh)
return (self.calc_false_positive_area_,
self.scurve_threshhold_error_)
>>> r = 4; b =16
>>> scurve_opt = SCurveOpt(target_s_thresh=0.5)
>>> scurve_opt(r,b)
(0.107047, 0.032862)
def combine_outputs(x, alpha=1.0):
area, thres_error = x
return (1-alpha)*area + alpha*thres_error
def opt(max_hash_budget, target_s_threshold,
alpha, use_s_thresh_approx, slices):
# Setup function
scurve = SCurveOpt(target_s_threshold, use_s_thresh_approx)
func = lambda x : combine_outputs(scurve(x[0], x[1]), alpha) \
if x[0]*x[1] <= max_hash_budget else np.inf
# Perform optimisation
x0 , fval, grid, Jout = scipy.optimize.brute(
func, slices, full_output=True, finish=None)
return x0 , fval, grid, Jout
Opt. args: [ 5. 26.] # Rows, Bands
[1] | "Adaptive Product Normalization: Using Online Learning for Record Linkage in Comparison Shopping"—Mikhail Bilenko, Sugato Basu & Mehran Sahami. |
[2] | "Locality-Sensitive Hashing for Finding Nearest Neighbors"—Malcolm Slaney & Michael Casey. |
[3] | Mining of Massive Datasets—J. Leskovec, A. Rajaraman, J. Ullman, http://www.mmds.org |